Content
Introduction to Probability
Probability refers to potential. A random event’s occurrence is the subject of this area of mathematics. The range of the value is 0 to 1. Mathematics has included probability to forecast the likelihood of certain events. The degree to which something is likely to happen is basically what probability means.
We will understand the potential outcomes for a random experiment using this fundamental theory of probability, which is also applied to the probability distribution. Knowing the total number of outcomes is necessary before we can calculate the likelihood that a certain event will occur.
Looking for last minute help for your AP Statistics exam in May? Find an expert 1-on-1 online AP Statistics tutor from Wiingy and give your exam prep a boost!
What is probability?
The probability serves as a gauge for how likely an event is to occur. It measures how likely an occurrence is.
In terms of quantitative measurements ranging from zero to one, probability theory offers a way to estimate the possibility of occurrences of various occurrences coming from a random experiment. A definite occurrence has a probability of one while an impossible event has a probability of zero.
Basic concepts of probability
- Sample space and events
A sample space is a set of potential results from a random experiment. The letter “S” is used to denote the sample space. Events are the subset of potential experiment results.
Discrete or finite sample spaces are those that have a finite number of outcomes. The set of all the possible outcomes of the random experiment can be considered to be the sample space.
Any subset of the sample space is and is known as an event.
- Probability of an event (P(A))
For an experiment, the probability is the chance of the occurrence of a particular event.
Taking (P(A)) to be the probability of the event A, then
Some properties of probability are as follows:
1.
2. if A is an impossible event.
3. if A is a sure event.
- Complementary events
When there are just two outcomes, complementary events take place.
A’ stands for the complimentary event of A, and for every event A, there is an equivalent event A’ that displays the other components of the sample space S.
Events A and A’ are mutually exhaustive and exclusive.
A’+A=S.
- Union of events
The set that contains every element from each set is referred to as the union of two or more sets. If an element belongs to at least one of the sets, it is said to be in the union. The word “or” is frequently used in conjunction with the sign representing the union. The reason for this is that AB is the collection of all components in A, B, or even both.
We list the components of sets A or B, or both, in order to determine the union of the two sets. The union of sets A and B in a Venn diagram may be expressed as the intersection of two completely shaded circles.
- Intersection of events
The set of items that are common to all two or more sets is referred to as their intersection. The intersection symbol and its association with the word “and” is used. This is so because AB is the collection of elements that both relate to A and B at the same time.
Only those components must be included whose listing appears in both or all of the sets in order to identify the intersection of two or more sets. The Venn diagram may be used to demonstrate this simply. Here, the shaded area may serve as a depiction of the intersection of two sets, A and B. This area may be at the centre of two concentric rings.
Probability rules and axioms
- Addition rule for mutually exclusive events
When two events are mutually exclusive the specific addition rule is valid. It claims that the probability of either event happening is equal to the probability of each event happening individually. If there are two events A and B which are mutually exclusive then the probability of event A or event B occurring id given by formula and is also equal to
and equal to
.
- Addition rule for non-mutually exclusive events
When two events A and B are non-mutually exclusive it means that there is some overlap between these events. The probability that A or B will occur is the sum of the probability of each event and the difference between the calculated sum and the probability of the overlap.
- Multiplication rule for independent events
If there are two independent events A and B then is equal to
.
- Multiplication rule for dependent events
Dependent events are ones in which the outcome of one event has an impact on the outcome of the other. Sometimes, the likelihood of the second occurrence depends on whether the first event occurs. The formula we use is .
Discrete probability distributions
A probability distribution that indicates the likelihood that a discrete random variable will have a certain value is known as a discrete probability distribution. Such a distribution will show data with a limited number of outcomes that may be counted. A discrete probability distribution has to meet two requirements which are as follows:
1. The probability that the discrete random variable is equal to
,lies between 0 and 1, i.e.,
.
2. The sum of all the probabilities is 1.
Some examples of discrete probability distributions are Poisson, Bernoulli’s, binomial, and geometric.
- Poisson: The discrete probability distribution known as the Poisson distribution is often employed in the banking industry. It provides the likelihood that a specific number of events will occur within a defined time frame.
- Notation:
PMF:
3. Bernoulli’s: A discrete probability distribution known as a Bernoulli distribution has a random variable that can either be equal to 0 (failure) or equal to 1. (success). The likelihood of success is , while the likelihood of failure is
.
Notation:
PMF:
4. Binomial: A discrete probability distribution called a binomial distribution provides the likelihood thatBernoulli trials will succeed. The value of
indicates the likelihood of success.
Notation:
PMF:
5. Geometric: Another kind of discrete probability distribution is called a geometric distribution, and it shows the likelihood of a series of failures before the first success.
Notation:
PMF: .
- Mean of the discrete probability distribution
The weighted average of all potential values for a discrete random variable is represented by the mean of the discrete probability distribution. It also goes by the name “expected value.” The following is the formula for a discrete random variable’s mean:
- Variance and standard deviation of the discrete probability distribution
The variance of the discrete probability distribution reveals the distribution’s dispersion around the mean. It may be described as the average of the squared deviations from the mean of the distribution,. The following is the formula for a discrete random variable’s variance:
Standard deviation is just the square root of the variance which is .
Continuous probability distribution
A random variable with an unlimited number of potential values is referred to as a continuous random variable. As a result, there is no chance that a continuous random variable will have an exact value of 0. A continuous random variable’s features are described using the probability density function and the cumulative distribution function.
There are mainly two types of continuous probability distribution.
1. Uniform random variable: A uniform random variable is a continuous random variable that describes a uniform distribution. Event probabilities are described by such a distribution.
PDF:
2. Normal random variable: A normal random variable is a continuous random variable that simulates a normal distribution.
Notations:
PDF:
- Mean of the continuous probability distribution
The weighted average value of the random variable, , can be used to define the mean of a continuous random variable. The continuous random variable’s expectation is another name for it. The formula is as follows:
- Variance and standard deviation of the continuous probability distribution
The expectation of the squared deviations from the mean may be used to define the variance of a continuous random variable. It aids in figuring out the continuous random variable’s distribution’s dispersion relative to the mean. The formula is as follows:.
Since the standard deviation is the square root of variance the formula is:
Conditional probability and independence
The potential of an event or result occurring dependent on the existence of a prior event or outcome is known as conditional probability. It is determined by multiplying the likelihood of the earlier occurrence by the increased likelihood of the later, or conditional, event.
- Multiplication rule for independent events and dependent events
According to the probability multiplication rule, the likelihood of both events A and B occurring is equal to the product of the probability of B occurring and the conditional probability of event A occurring if event B occurs.
- Bayes’ theorem
Solved examples
Example 1: If a box contains 3 blue balls and 5 yellow balls, what is the probability of picking up a yellow ball? Also, find the probability of picking a blue ball.
Solution 1:
The probability of picking a yellow ball using the formula ,
We get a number of outcomes favourable to picking a yellow ball is 5.
Therefore,
To calculate the probability of picking a blue ball, we see the number of outcomes favourable to picking a yellow ball is 3.
Therefore,
Example 2: There is a class of 20 students. The teacher wants to pick 5 students for an assignment. What is the probability that a student named X( only one in class) gets chosen.
Solution 2:
The probability of X being chosen is the total number of ways in which the group of students can be selected with X in it divided by the total number of possible groups.
The possible total number of groups would be choosing 5 students out of 20 which would be
The ways in which X would be chosen would be when there is only 4 other kids left to choose which would be
Now finding the probability of X being chosen is
Example 3: A bag has 4 blue balls and 4 red balls. What is the probability of picking 2 blue balls?
Solution 3:
The probability of picking the first blue ball is
The probability of picking the second blue ball from the leftover balls is .
Therefore, the probability of picking 2 blue balls is
Example 4: The probability of two students solving a problem independently is and
respectively. Calculate the probability that the problem will be solved.
Solution 4:
Since the probabilities of them solving are independent of each other =
And the probability the problem can be solved is given by
Example 5: If an unbiased die is thrown thrice what is the probability of the sum of all three throws being 8.
Solution 5:
The sum can be 8 in the following cases:
Which is 21 cases out of cases
Therefore, the probability of getting a 8 is
Conclusion
In this article, we learnt about probability and how to sample space and events used in the calculation of probability. We also learnt about the rules and axioms of probability and about discrete probability distributions, continuous probability distribution and conditional probability and independence.
Looking for last minute help for your AP Statistics exam in May? Find an expert 1-on-1 online AP Statistics tutor from Wiingy and give your exam prep a boost!
Frequently asked questions (FAQs)
What is PDF in statistics?
The probability density function (PDF), is a statistical statement that describes a probability distribution of a discrete random variable.
What is PMF in statistics?
The likelihood that a discrete random variable would exactly equal a certain value is expressed by a function called the probability mass function.
What are dependent events?
Occurrences that are dependent upon prior events are called dependent events.
What are independent events?
Events classified as independent do not depend on other events for their occurrence.
What is an impossible event indicate?
It indicates that the event can never occur because it was never part of the sample space.
References
- Grimmett, G., & Welsh, D. (2014). Probability: an introduction. Oxford University Press
- Ross, S. M. (2014). Introduction to probability models. Academic Press
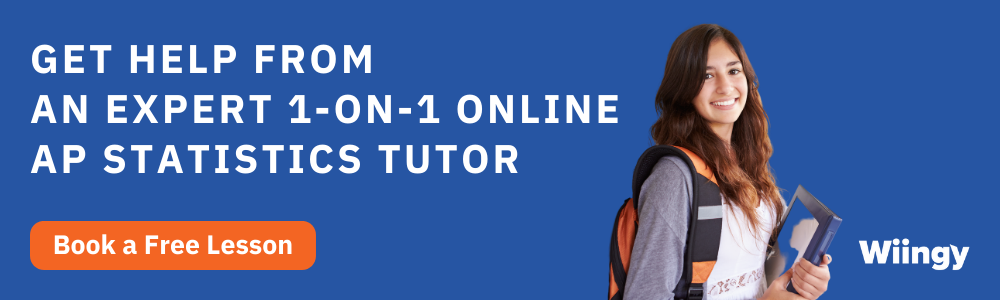
Written by
Prerit Jain